Notice
Recent Posts
Recent Comments
Link
일 | 월 | 화 | 수 | 목 | 금 | 토 |
---|---|---|---|---|---|---|
1 | 2 | 3 | 4 | 5 | ||
6 | 7 | 8 | 9 | 10 | 11 | 12 |
13 | 14 | 15 | 16 | 17 | 18 | 19 |
20 | 21 | 22 | 23 | 24 | 25 | 26 |
27 | 28 | 29 | 30 | 31 |
Tags
- 통계
- 자격증
- 파이썬
- 데이터 전처리
- pytorch
- 시각화
- r
- SQL
- sklearn
- 코딩테스트
- 데이터 분석
- 머신러닝
- 태블로
- Deep Learning Specialization
- Google ML Bootcamp
- tableau
- Python
- scikit learn
- 딥러닝
- matplotlib
- IRIS
- 데이터분석준전문가
- SQLD
- pandas
- 데이터분석
- 이것이 코딩테스트다
- ADsP
- 회귀분석
- 이코테
- ML
Archives
- Today
- Total
함께하는 데이터 분석
[Find-A][Pytorch] 학습률 본문
학습률
적절한 모델, 적절한 초깃값을 설정했음에도 학습률에 따라 모델의 학습이 달라짐
학습률은 손실에 대한 가중치를 구하고 그 값과 학습률을 곱해서 변수들을 업데이터 하는데에 사용됨
학습률이 너무 높다면 업데이트 방향이 맞아도 너무 크게 업데이트 되고, 너무 낮다면 지엽적인 공간에서의 극솟값에만 도달하므로 전체 손실 공간에서의 극솟값에 도달할 수 없게 됨
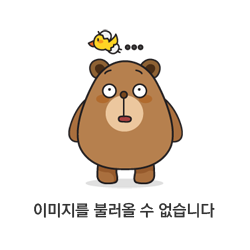
따라서 적절한 학습률을 찾아야 모델이 학습을 잘하고 전체 손실 공간에서 극솟값을 찾을 수 있음
보통 실무에서는 초기에 비교적 높은 학습률로 시작하여 점차 학습률을 낮추는 전략을 취함
하지만 이 방법이 정석은 아니고 오히려 배치 사이즈를 늘리는 게 더 좋다는 연구도 있음
학습률을 점차 떨어뜨리는 방법을 학습률 부식이라고 하는데 이와 관련된 파이토치 함수들은 torch.optim에 구현되어 있음
Pytorch
라이브러리 불러오기
import numpy as np
import torch
import torch.nn as nn
import torch.optim as optim
import torch.nn.init as init
import torchvision
import torchvision.datasets as dset
import torchvision.transforms as transforms
from torch.optim import lr_scheduler
from torch.utils.data import DataLoader
batch_size = 256
learning_rate = 0.001
num_epoch = 10
Data
mnist_train = dset.MNIST("./", train=True, transform=transforms.ToTensor(), target_transform=None, download=True)
mnist_test = dset.MNIST("./", train=False, transform=transforms.ToTensor(), target_transform=None, download=True)
print(mnist_train.__getitem__(0)[0].size(), mnist_train.__len__())
mnist_test.__getitem__(0)[0].size(), mnist_test.__len__()
>>> (torch.Size([1, 28, 28]), 10000)
train_loader = torch.utils.data.DataLoader(mnist_train,batch_size=batch_size, shuffle=True,num_workers=2,drop_last=True)
test_loader = torch.utils.data.DataLoader(mnist_test,batch_size=batch_size, shuffle=False,num_workers=2,drop_last=True)
CNN Model
class CNN(nn.Module):
def __init__(self):
super(CNN,self).__init__()
self.layer = nn.Sequential(
nn.Conv2d(1,16,3,padding=1), # 28 x 28
nn.ReLU(),
nn.Conv2d(16,32,3,padding=1), # 28 x 28
nn.ReLU(),
nn.MaxPool2d(2,2), # 14 x 14
nn.Conv2d(32,64,3,padding=1), # 14 x 14
nn.ReLU(),
nn.MaxPool2d(2,2) # 7 x 7
)
self.fc_layer = nn.Sequential(
nn.Linear(64*7*7,100),
nn.ReLU(),
nn.Linear(100,10)
)
def forward(self,x):
out = self.layer(x)
out = out.view(batch_size,-1)
out = self.fc_layer(out)
return out
Loss func & Optimizer
USE_CUDA = torch.cuda.is_available() # gpu 사용 가능하면 true or false
device = torch.device("cuda" if USE_CUDA else "cpu")
print(f'다음 기기로 학습 : {device}')
>>> 다음 기기로 학습 : cpu
model = CNN().to(device)
loss_func = nn.CrossEntropyLoss()
optimizer = torch.optim.SGD(model.parameters(), lr=learning_rate)
# 지정한 스텝 단위로 학습률에 감마를 곱해 학습률을 감소시킵니다.
#scheduler = lr_scheduler.StepLR(optimizer, step_size=1, gamma= 0.99)
# 지정한 스텝 지점(예시에서는 10,30,80)마다 학습률에 감마를 곱해줍니다.
#scheduler = lr_scheduler.MultiStepLR(optimizer, milestones=[10,30,80], gamma= 0.1)
# 매 epoch마다 학습률에 감마를 곱해줍니다.
#scheduler = lr_scheduler.ExponentialLR(optimizer, gamma= 0.99)
# https://pytorch.org/docs/stable/optim.html?highlight=lr_scheduler#torch.optim.lr_scheduler.ReduceLROnPlateau
# 지정한 메트릭으로 측정한 값이 더 나아지지 않으면 학습률을 감소시킵니다. ex) 정확도, dice score 등등
# 이 스케쥴러에는 다양한 인자가 들어가는데 각각의 역할은 도큐먼트를 참고 바랍니다.
# 여기서는 patience 즉, 지정한 값이 줄어들지 않을때 몇 epoch 만큼을 지켜볼 것인지를 1로 낮춰놨기 때문에 매 epoch 마다 학습률이 감소하는것을 확인할 수 있습니다.
scheduler = lr_scheduler.ReduceLROnPlateau(optimizer,threshold=1,patience=1,mode='min')
# 참고 https://www.geeksforgeeks.org/python-dir-function/
print(dir(scheduler))
print(dir(optimizer))
>>> ['__class__', '__delattr__', '__dict__', '__dir__', '__doc__', '__eq__', '__format__', '__ge__', '__getattribute__', '__gt__', '__hash__', '__init__', '__init_subclass__', '__le__', '__lt__', '__module__', '__ne__', '__new__', '__reduce__', '__reduce_ex__', '__repr__', '__setattr__', '__sizeof__', '__str__', '__subclasshook__', '__weakref__', '_init_is_better', '_reduce_lr', '_reset', 'best', 'cooldown', 'cooldown_counter', 'eps', 'factor', 'in_cooldown', 'is_better', 'last_epoch', 'load_state_dict', 'min_lrs', 'mode', 'mode_worse', 'num_bad_epochs', 'optimizer', 'patience', 'state_dict', 'step', 'threshold', 'threshold_mode', 'verbose']
['__class__', '__delattr__', '__dict__', '__dir__', '__doc__', '__eq__', '__format__', '__ge__', '__getattribute__', '__getstate__', '__gt__', '__hash__', '__init__', '__init_subclass__', '__le__', '__lt__', '__module__', '__ne__', '__new__', '__reduce__', '__reduce_ex__', '__repr__', '__setattr__', '__setstate__', '__sizeof__', '__str__', '__subclasshook__', '__weakref__', '_cuda_graph_capture_health_check', '_hook_for_profile', '_warned_capturable_if_run_uncaptured', '_zero_grad_profile_name', 'add_param_group', 'defaults', 'load_state_dict', 'param_groups', 'state', 'state_dict', 'step', 'zero_grad']
Train
for i in range(num_epoch):
# ReduceLRONPlateau 빼고는 아래의 코드를 사용하세요
#scheduler.step()
for j,[image,label] in enumerate(train_loader):
x = image.to(device)
y_= label.to(device)
optimizer.zero_grad()
output = model.forward(x)
loss = loss_func(output,y_)
loss.backward()
optimizer.step()
# ReduceLRONPlateau 만 해당됩니다. 이 코드에서는 손실이 줄어들지 않으면 학습률을 낮추도록 만들어놨습니다.
scheduler.step(loss)
if i % 10 == 0:
print(loss)
#print("Epoch: {}, Learning Rate: {}".format(i,scheduler.get_lr()))
print("Epoch: {}, Learning Rate: {}".format(i,scheduler.optimizer.state_dict()['param_groups'][0]['lr']))
>>> tensor(2.3034, grad_fn=<NllLossBackward0>)
Epoch: 0, Learning Rate: 0.001
Epoch: 1, Learning Rate: 0.0001
Epoch: 2, Learning Rate: 0.0001
Epoch: 3, Learning Rate: 1e-05
Epoch: 4, Learning Rate: 1e-05
Epoch: 5, Learning Rate: 1.0000000000000002e-06
Epoch: 6, Learning Rate: 1.0000000000000002e-06
Epoch: 7, Learning Rate: 1.0000000000000002e-07
Epoch: 8, Learning Rate: 1.0000000000000002e-07
Epoch: 9, Learning Rate: 1.0000000000000004e-08
Test
correct = 0
total = 0
with torch.no_grad():
for image,label in test_loader:
x = image.to(device)
y_= label.to(device)
output = model.forward(x)
_,output_index = torch.max(output,1)
total += label.size(0)
correct += (output_index == y_).sum().float()
print("Accuracy of Test Data: {}".format(100*correct/total))
>>> Accuracy of Test Data: 9.805688858032227
https://www.hanbit.co.kr/store/books/look.php?p_code=B7818450418
파이토치 첫걸음
딥러닝 구현 복잡도가 증가함에 따라 ‘파이써닉’하고 사용이 편리한 파이토치가 주목받고 있다. 파이토치 코리아 운영진인 저자는 다년간 딥러닝을 공부하고 강의한 경험을 살려 딥러닝의
www.hanbit.co.kr
'학회 세션 > 파인드 알파' 카테고리의 다른 글
[Find-A] 생성적 적대 신경망 (0) | 2022.10.02 |
---|---|
[Find-A][Pytorch] 정규화 (1) | 2022.09.25 |
[Find-A][Pytorch] 초기화 (0) | 2022.09.25 |
[Find-A][Pytorch] 임베딩 함수를 이용한 LSTM, GRU 모델 구현 (1) | 2022.09.21 |
[Find-A] LSTM, GRU, 임베딩 (1) | 2022.09.21 |