Notice
Recent Posts
Recent Comments
Link
일 | 월 | 화 | 수 | 목 | 금 | 토 |
---|---|---|---|---|---|---|
1 | ||||||
2 | 3 | 4 | 5 | 6 | 7 | 8 |
9 | 10 | 11 | 12 | 13 | 14 | 15 |
16 | 17 | 18 | 19 | 20 | 21 | 22 |
23 | 24 | 25 | 26 | 27 | 28 |
Tags
- pandas
- Python
- 이것이 코딩테스트다
- 데이터 전처리
- Deep Learning Specialization
- matplotlib
- r
- 회귀분석
- 머신러닝
- 딥러닝
- SQL
- scikit learn
- 데이터분석준전문가
- tableau
- 통계
- 데이터 분석
- SQLD
- 태블로
- 이코테
- 자격증
- 파이썬
- 데이터분석
- ADsP
- 코딩테스트
- 시각화
- pytorch
- ML
- IRIS
- Google ML Bootcamp
- sklearn
Archives
- Today
- Total
함께하는 데이터 분석
[Python] Pandas - ② 본문
저번에 다 작성하지 못했던 Pandas 라이브러리를 마무리하려고 합니다!
그럼 시작해볼까요?
5-1. 결측치 여부 확인
df2.isnull()
df2.isnull().sum() # 각 열마다 결측치 개수 출력
>>> Name 0
Age 0
Score 0
Score2 2
dtype: int64
5-2. 결측치가 존재하는 행 삭제
df2.dropna(how = 'any') # how = 'all' : 행의 모든 값이 NaN인 경우 삭제
5-3. 결측치 대체
df2.fillna(value = 50.0) # 기본적으로 저장 X
df2['Score2'].fillna({'two' : 68.0, 'five': 80.0}, inplace = True) # inplace = True : 저장
df2
6-1. 기술 통계
기본적으로 NaN 값은 배제하고 계산한다.
df2.sum(axis = 0) # 행 방향으로의 합 (각 열의 합), default
>>> Name KimChoiLeeParkKimShin
Age 105
Score 520.5
Score2 464.5
dtype: object
df2.sum(axis = 1) # 열 방향으로의 합 (각 행의 합)
>>> one 179.0
two 165.0
three 194.0
four 183.0
five 187.0
six 182.0
dtype: float64
sum() | 합계 |
mean() | 평균 |
median() | 중위수 |
var() | 분산 |
std() | 표준편차 |
count() | 갯수 (NaN 제외) |
min() | 최소값 |
max() | 최대값 |
cumsum() | 누적합 |
cumprod() | 누적곱 |
# 상관계수
df2['Score'].corr(df2['Score2'])
>>> 0.49326856034623434
#공분산
df2['Score'].cov(df2['Score2'])
>>> 17.575
6-2. apply : 함수 적용하기
df3 = pd.DataFrame(np.random.randn(4, 3), columns = ["A", "B", "C"])
df3
df3.apply(np.cumsum) # 누적합
# 사용자 정의 함수 이용
func = lambda x: x.max() - x.min()
df3.apply(func, axis = 0)
>>> A 1.508441
B 2.406838
C 1.605722
dtype: float64
6-3. 빈도 구하기
df2['Name'].value_counts()
>>> Kim 2
Park 1
Lee 1
Shin 1
Choi 1
Name: Name, dtype: int64
7-1. index 기준 정렬
# index가 오름차순이 되도록 정렬
df2.sort_index(axis = 0) # default : ascending = True
# column을 기준으로 내림차순이 되도록 정렬
df2.sort_index(axis = 1, ascending = False)
7-2. 값 기준 정렬
# 'Age' 열의 값을 기준으로 오름차순 정렬
df2.sort_values(by = 'Age') # default : ascending = True (오름차순)
# 두 개 이상의 column을 기준으로 내림차순 정렬
df2.sort_values(by = ['Age', 'Score'], ascending = False)
8. 그룹화
df = pd.DataFrame({
'group1': ['F', 'M', 'F', 'M', 'F', 'M', 'F', 'F'],
'group2': ['a', 'a', 'b', 'c', 'b', 'b', 'a', 'c'],
'score1': np.random.randint(0, 10, size = 8),
'score2': np.random.randint(0, 10, size = 8)
})
df
# group1별 합계 구하기
df.groupby('group1').sum()
# group1, group2별 평균 구하기
df.groupby(['group1', 'group2']).mean()
9. 데이터 입출력
df = pd.DataFrame(np.random.rand(6, 4), index = pd.date_range('20210122', periods = 6), columns = ['A', 'B', 'C', 'D'])
df
# 데이터프레임을 csv 형식으로 저장하기
df.to_csv('df_ex.csv', header = True, index = False) # column명 가져오고 index명 가져오지 않음
# csv 형식으로 된 파일 불러오기
df_ex = pd.read_csv('df_ex.csv')
df_ex
여기까지 입니다.
감사합니다!!
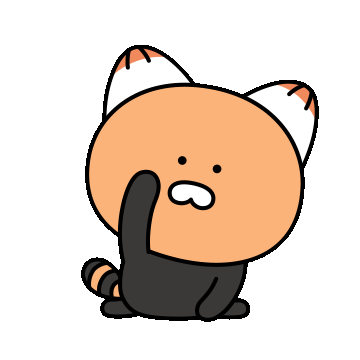
'데이터분석 공부 > Python' 카테고리의 다른 글
[Python] Seaborn을 활용한 범주형 변수의 시각화 (0) | 2022.03.19 |
---|---|
[Python] Seaborn을 활용한 수치형 변수의 시각화 (0) | 2022.03.18 |
[Python] Pandas - ① (0) | 2022.01.24 |
[Python] NumPy (0) | 2022.01.23 |
[Python] Jupyter Notebook 설치 및 실행 (0) | 2022.01.22 |