일 | 월 | 화 | 수 | 목 | 금 | 토 |
---|---|---|---|---|---|---|
1 | ||||||
2 | 3 | 4 | 5 | 6 | 7 | 8 |
9 | 10 | 11 | 12 | 13 | 14 | 15 |
16 | 17 | 18 | 19 | 20 | 21 | 22 |
23 | 24 | 25 | 26 | 27 | 28 |
- tableau
- 이코테
- 이것이 코딩테스트다
- 데이터분석준전문가
- 머신러닝
- 코딩테스트
- pytorch
- SQL
- ADsP
- 통계
- IRIS
- 데이터분석
- 자격증
- 딥러닝
- Python
- 회귀분석
- scikit learn
- r
- 시각화
- ML
- SQLD
- pandas
- 파이썬
- Google ML Bootcamp
- 데이터 분석
- 데이터 전처리
- sklearn
- Deep Learning Specialization
- matplotlib
- 태블로
- Today
- Total
목록의사결정 나무 (2)
함께하는 데이터 분석
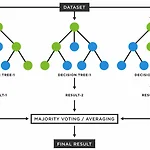
랜덤 포레스트(Random Forest) 배깅 방식을 적용한 의사결정 나무(Decision Tree)의 앙상블 따라서 사이킷런의 BaggingClassifier에 DecisionTreeClassifier를 넣어 만들거나 RandomForestClassifier를 사용 사이킷런 from sklearn.model_selection import train_test_split from sklearn.datasets import make_moons X, y = make_moons(n_samples=500, noise=0.3, random_state=42) X_train, X_test, y_train, y_test = train_test_split(X, y, random_state=42) 사이킷런의 moons 데이..
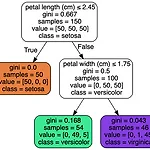
의사결정 나무(Decision Tree) 분류와 회귀 작업, 다중출력 작업도 가능한 다재다능한 머신러닝 알고리즘 최근에 자주 사용되는 강력한 머신러닝 알고리즘 중 하나인 랜덤 포레스트의 기본 구성 요소 1. 의사결정 나무 학습과 시각화 from sklearn.datasets import load_iris from sklearn.tree import DecisionTreeClassifier iris = load_iris() X = iris['data'][:, (2, 3)] y = iris['target'] 사이킷런의 iris 데이터를 불러오고 X에 PetalLength, PetalWidth y에 꽃의 품종인 Setona, Versicolor, Virginica를 할당 tree_clf = DecisionTr..