일 | 월 | 화 | 수 | 목 | 금 | 토 |
---|---|---|---|---|---|---|
1 | 2 | 3 | 4 | 5 | 6 | 7 |
8 | 9 | 10 | 11 | 12 | 13 | 14 |
15 | 16 | 17 | 18 | 19 | 20 | 21 |
22 | 23 | 24 | 25 | 26 | 27 | 28 |
29 | 30 |
- Python
- 이코테
- pandas
- 코딩테스트
- matplotlib
- 데이터분석
- 자격증
- ML
- 시각화
- scikit learn
- 데이터분석준전문가
- sklearn
- tableau
- 데이터 전처리
- Deep Learning Specialization
- IRIS
- 이것이 코딩테스트다
- 딥러닝
- SQL
- 데이터 분석
- r
- 회귀분석
- pytorch
- 태블로
- SQLD
- 파이썬
- ADsP
- 통계
- 머신러닝
- Google ML Bootcamp
- Today
- Total
목록Neural Networks and Deep Learning (4)
함께하는 데이터 분석
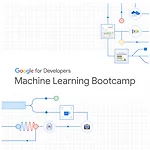
Deep neural network notation Forward propagation in a deep network Parameters W[l] and b[l] Vectorized implementation Intuition about deep representation Forward and Backward fuctions Forward propagation for layer l Backward propagation for layer l What are hyperparameters?
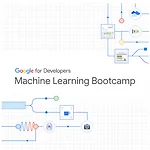
Neural Network Representation Computing a Neural Network's Output Vectorizing across multiple examples Justification for vectorized implementation Activation functions Why do you need Non-Linear Activation Functions? Derivatives of Activation Functions Gradient descent for neural networks Formulas for computing derivatives What happens if you initialize weights to zero? Random initialization
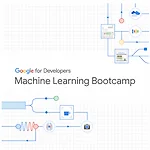
Linear Regression Logistic Regression Logistic Regression cost function Gradient Descent Logistic Regression Gradient Descent Logistic Regression Gradient Descent on m examples Vectorization import numpy as np import time a = np.random.rand(1000000) b = np.random.rand(1000000) tic = time.time() c = 0 for i in range(1000000) : c += a[i] * b[i] toc = time.time() print('for loop :' + str(1000 * (to..