일 | 월 | 화 | 수 | 목 | 금 | 토 |
---|---|---|---|---|---|---|
1 | ||||||
2 | 3 | 4 | 5 | 6 | 7 | 8 |
9 | 10 | 11 | 12 | 13 | 14 | 15 |
16 | 17 | 18 | 19 | 20 | 21 | 22 |
23 | 24 | 25 | 26 | 27 | 28 |
- r
- tableau
- 회귀분석
- Google ML Bootcamp
- sklearn
- scikit learn
- 머신러닝
- pytorch
- ADsP
- 통계
- 데이터분석준전문가
- matplotlib
- ML
- 태블로
- 데이터분석
- Python
- 시각화
- Deep Learning Specialization
- 파이썬
- 딥러닝
- SQLD
- 이코테
- 이것이 코딩테스트다
- SQL
- 데이터 전처리
- IRIS
- pandas
- 코딩테스트
- 데이터 분석
- 자격증
- Today
- Total
목록전체 글 (142)
함께하는 데이터 분석
from sklearn.ensemble import RandomForestClassifier from sklearn.tree import DecisionTreeClassifier from sklearn.linear_model import LogisticRegression from xgboost import XGBClassifier from sklearn.naive_bayes import GaussianNB from sklearn.neighbors import KNeighborsClassifier rfc = RandomForestClassifier() dtc = DecisionTreeClassifier() lrc = LogisticRegression(solver='liblinear') xgb = XGBCl..
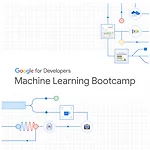
Deep neural network notation Forward propagation in a deep network Parameters W[l] and b[l] Vectorized implementation Intuition about deep representation Forward and Backward fuctions Forward propagation for layer l Backward propagation for layer l What are hyperparameters?
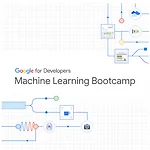
Neural Network Representation Computing a Neural Network's Output Vectorizing across multiple examples Justification for vectorized implementation Activation functions Why do you need Non-Linear Activation Functions? Derivatives of Activation Functions Gradient descent for neural networks Formulas for computing derivatives What happens if you initialize weights to zero? Random initialization
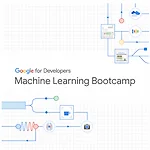
Linear Regression Logistic Regression Logistic Regression cost function Gradient Descent Logistic Regression Gradient Descent Logistic Regression Gradient Descent on m examples Vectorization import numpy as np import time a = np.random.rand(1000000) b = np.random.rand(1000000) tic = time.time() c = 0 for i in range(1000000) : c += a[i] * b[i] toc = time.time() print('for loop :' + str(1000 * (to..
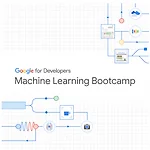
Supervised Learning with Neural Networks Neural Network examples Scaled drives deep learning progress
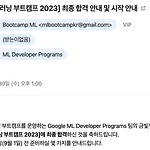
이번 2023 구글 머신러닝 부트캠프에 붙어서 부트캠퍼로 참여하게 되었습니다 지원하게 된 계기는 취업을 준비하면서 공부했던 내용을 정리하면서 복습하고 싶었는데 딱 맞을 것 같아서 지원했습니다 프로그램 구성 프로그램 일정 참가 회사 합격 후기 지원하게 된 가장 큰 이유가 Andrew Ng 교수의 Deep Learning Specialization 수업을 듣고 정리하는 것이기에 강의를 듣고 공부한 부분을 시간 관계상 설명까지 추가하여 올리진 못하더라도 나름의 정리를 해서 올릴 예정입니다
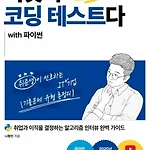
음료수 얼려 먹기 n, m = map(int, input().split()) graph = [] for i in range(n) : graph.append(list(map(int, input()))) def dfs(x, y) : if x = n or y = m : return False if graph[x][y] == 0 : graph[x][y] = 1 dfs(x - 1, y) dfs(x + 1, y) dfs(x, y - 1) dfs(x, y + 1) return True return False result = 0 for i in range(n) : for j in range(m) : if dfs(i, j) == True : result += 1 print(result..
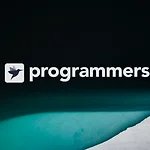
최댓값과 최솟값 def solution(s): answer = list(map(int,s.split())) return str(min(answer)) + ' ' + str(max(answer)) JadenCase 문자열 만들기 def solution(s): answer = list(map(str, s.split(' '))) for i in range(len(answer)) : answer[i] = answer[i].capitalize() return ' '.join(answer) 최솟값 만들기 def solution(A, B) : A.sort() B.sort(reverse = True) summ = 0 for i in range(len(A)) : summ += A[i] * B[i] return summ ..
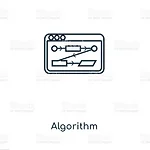
소수 판별(에라토스테네스의 체) import math n = 1000 array = [True for i in range(n + 1)] array[1] = 0 for i in range(1, int(math.sqrt(n)) + 1) : if array[i] == True : j = 2 while i * j 0 : num, mod = divmod(num, n) rev_base += str(mod) return rev_base[::-1] 1에서 n까지 각 수의 약수의 개수 import math n = 15 arr = [] for i in range(1, n + 1) : count = 0 for j in range(1, int(math.sqrt(i)) + 1) : if j * j == i : count += ..
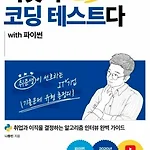
부품 찾기 # 부품 찾기 (시간 초과할 수도) N = int(input()) arr = list(map(int, input().split())) M = int(input()) data = list(map(int, input().split())) for i in range(len(data)) : if data[i] in arr : print('yes', end = ' ') else : print('no', end = ' ') # 부품 찾기(이진 탐색) def binary_search(array, target, start, end) : while start target : # 중간점보다 target이 작으므로 왼쪽 end = mid - 1 else : # 중간점보다 target이 크므로 오른쪽 start =..